Learn about infections, sepsis, and the immune system as an ideal diagnostic
Acute Infection and Sepsis
Visits to the emergency department due to acute infection symptoms are common
Acute infections are extremely common. When their symptoms are sufficiently severe or access to care elsewhere is not available, patients visit the emergency department for diagnosis and treatment. We estimate over 20 million and 15% of all ED visits relate to symptoms of acute infections.1
Patients coming to the emergency department are presumed ill until proven otherwise. Patients suspected of infection are no different. As a result, physicians usually assess how ill is the patient and whether the illness is due to an acute infection.
A diagnosis requires both insights.
Typical approach used to assess patients coming to the emergency department2
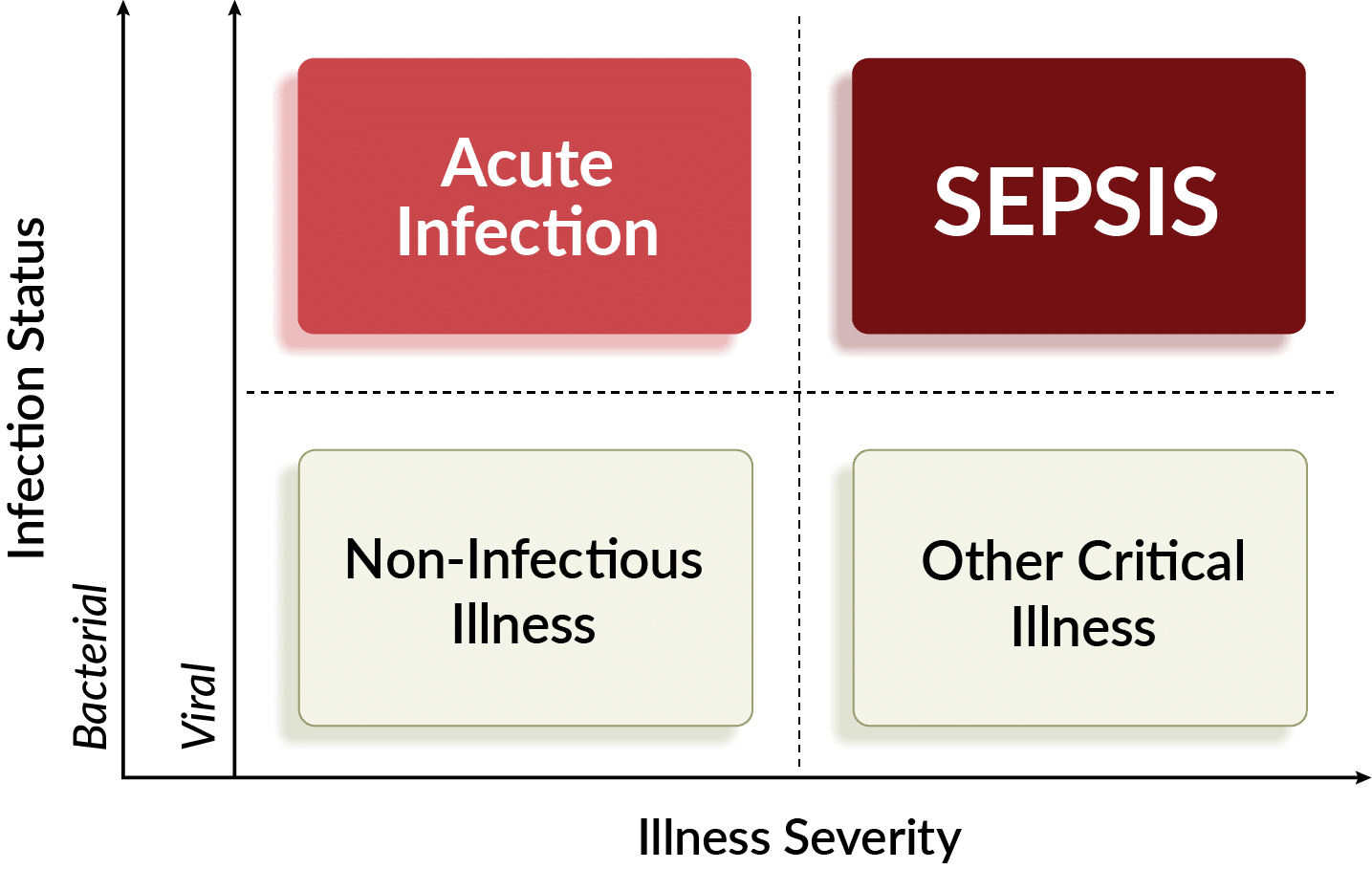
NON-INFECTIOUS illness & other critical illness
Infection and sepsis mimics are hard to diagnose and can be challenging to manage3,4,5
About 20% of non-severely ill patients with symptoms of infection do not actually have one. Their symptoms may by caused by allergy, asthma, non-severe COPD exacerbation, or other disease. Ruling out an infection and narrowing down the actual cause of illness can be important to provide peace of mind, reduce resource utilization and limit escalation of disease.6
Representative Sepsis Mimics3,4,5
ACUTE INFECTION
Infected patients without sepsis are also challenging to diagnose in the Emergency Department
While sepsis is gravely concerning, most patients with infections in the ED do not have life threatening infection. Knowing whether an infection is bacterial or viral impacts antibiotic prescribing, discharge versus admission and other treatment decisions. Better tests that can more accurately rule-out bacterial infections may help reduce unnecessary antibiotic usage, which occurs an estimated 39% of the time.7
UNNECESSARY ANTIBIOTIC Rx
Patients receive antibiotics unnecessarily 39% of the time.7
Inappropriate antibiotic prescribing can have significant consequences as patients on antibiotics experience adverse events 20% of the time and excess antibiotic use risks an increase in antimicrobial resistance.8
Nevertheless, many are challenging to manage. In many patients, common infections such as those located in the respiratory, abdominal, skin and soft tissue, or urinary tract often do not yield positive results on routine pathogen tests. For example, in a study published in the New England Journal of Medicine of confirmed pneumonia patients, 62% did not have positive microbiology.9
SEPSIS
A concerning and dangerous subset of patients with acute infections
Sepsis is a dysregulated immune response that can be triggered by any acute infection. It can lead to tissue damage, organ failure, and worse. With the highest hospital mortality of any diagnosis, sepsis accounts for nearly half of in-hospital deaths. Identifying sepsis quickly is vital as risk of death increases by 7% for every hour in delay of antibiotics treatment.10
An estimated 1.7 million of the 20 million patients assessed for infection are diagnosed with sepsis.1,11,12 Given that over 270,000 die from sepsis, more than breast cancer, prostate cancer, and HIV/AIDS combined, it garners great urgency and focus by Emergency Medicine physicians and hospital staff.13
A fraction of septic patients are bacteremic
Only 10% of hospitalized patients have a bloodstream infection
Only 53% of septic patients have a bloodstream infection
Sepsis ≠ Bloodstream Infection
Contrary to widespread belief, bacteria is often not found in the blood of patients with acute infections or sepsis. Less than 10% of patients hospitalized for acute infections and only about half of patients with septic shock are “bacteremic” (have infection in the blood).14,15
IMMUNE RESPONSE
The Power of the Immune Response
Our immune system has developed targeted responses to different types of infections and other diseases over millions of years. Depending on the insult, the immune system will react differently. Its response to a serious bacterial infection is different than to a non-serious viral one or non-infectious reaction, like an allergic reaction.
Traditional immune markers have not been good enough
The clinical performance of traditional biomarkers like CRP, lactate, and PCT limits their ability to be reliable rule-in or rule-out tests. These general markers of inflammation or insult have been widely adopted given the need to determine the presence, type, and severity of infection. Nevertheless, unmet needs for more accurate tests, with better sensitivity and specificity performance, remain.
Host response opens new doors in the clinical setting
This century, the molecular revolution has included the ability to rapidly measure many markers at once (thanks to advances in lab techniques) and gain insights into what they mean (as a result of progress in bioinformatic techniques).
The combination of these two technological advancements promises to provide clinicians what they want: rapid tests that are highly accurate (high sensitivity and high specificity) that enable confident infectious disease presence and severity prognosis.
Explore how the immune system can address the challenges of diagnosing acute infections
General education website relating to the power of host response diagnostics to address unmet needs in diagnosing acute infection and sepsis in the emergency department.
MACHINE LEARNING
Transforming diagnostics with machine learning and artificial intelligence
Ingredients for a Successful ML Program
At Inflammatix ML, we understand that bringing world-class ID diagnostics to the point of care demands a holistic approach. Our highly interdisciplinary team draws on decades of experience in academic and industrial science with backgrounds ranging from applied statistics, computer science, engineering, bioinformatics, and software development. We pursue both methodological and applied research directions, developing novel models and methods when the need arises but also investigating the feasibility of the ‘tried-and-true’ as well as the ‘latest-and-greatest.’
We prioritize sharing our work and insights with the broader research community in both publications and international conferences. We continue to grow and curate one-of-a-kind patient datasets, leveraging information at multiple scales to guide selection of high-performing biomarkers and diagnostic classifiers. We engage stakeholders across the company and the medical community to help our test achieve leading performance and optimal integration with clinical workflows and decision-making.
Areas of Focus
Systems for diagnostic classifier development
Hyperparameter optimization of diagnostic classifiers
Fairness in diagnostics
Systems for diagnostic classifier development
Patient host response, as measured by expression of targeted mRNA biomarkers from blood, can vary from patient to patient. Previous approaches have developed host response signatures and classifiers that tend to generalize poorly, owing to biomarker selection and classifier training and validation on a limited, unrepresentative set of patient observations from a single study or hospital. Pooling of data across multiple studies has proven effective in producing more generalizable host response signatures and classifiers but introduces other methodological challenges.
Read more
For example, before being used in standard classifier development, our multi-cohort data must first be co-normalized to minimize spurious variation unrelated to our classification tasks. Also, the manner in which we select our classifiers must move beyond random cross-validation to reflect the structure and heterogeneity in our patient population and to provide more realistic estimates of generalization performance. In addition, our training data have been profiled with multiple assay platforms, none of which are fast enough to enable clinically actionable turnaround times for indications like sepsis.
Our biomarker signatures and classifiers must be able to generalize to measurements obtained on more deployment-ready platforms, possibly without access to such limited data at training time. We addressed these challenges in an important proof-of-concept study that helped systematize our process of diagnostic classifier development.
Hyperparameter optimization of diagnostic classifiers
An essential step in classifier selection is hyperparameter optimization, or the identification of values for a classifier’s hyperparameters that optimize some objective function (e.g., performance in cross-validation). While conventional methods of grid search and random sampling can still be effective, more sophisticated approaches such as Bayesian optimization16 and Hyperband17 have demonstrated gains in terms of both performance and efficiency (i.e., producing high-performing classifiers with fewer evaluations of candidate hyperparameterizations) on a range of ML benchmarks.
Read more
However, these ML studies tended to focus on computer vision tasks and to use large-scale and fairly homogeneous datasets, making their insights less applicable for our setting. Also, these studies only considered performance in internal validation (e.g., cross-validation or validation with a small, left-out subset of the training data), rarely if ever evaluating selected classifier performance in a completely different dataset (external validation). Moreover, such hyperparameter optimization studies in the healthcare domain have been limited. In a first-of-its-kind benchmarking analysis for molecular diagnostics, we compared the internal and external validation performance of classifiers selected by conventional approaches such as grid search and random sampling as well as Bayesian optimization.
Fairness in diagnostics
Recent studies have increasingly shown potential for performance disparities of deployed ML systems associated with demographic characteristics of the populations those systems are intended to serve. These disparities can have harmful downstream consequences when the system outputs are used to guide or replace human decision-making and can arise for reasons such as low representation of certain subgroups in training data or choices of features or training objectives that accentuate such disparities.
Read more
These important observations dovetail with decades of research in genetics and epidemiology on the potential confounding effects of demographic variation on associations between biological variables and clinical outcomes. Indeed, the biomarkers we select from multi-cohort data likely carry associations with demographic characteristics of different patient subpopulations that could have downstream impacts on the performance of our diagnostic classifiers. We are actively developing resources and techniques as part of an end-to-end framework to audit and mitigate subgroup disparities in our classifiers, ensuring equitable as well as high performance across our diverse patient population.
Want more details on our ML/AI progress?
INFLAMMATIX ADVOCACY
Committed to
Non-Profit and Government Partners
Inflammatix is committed to end sepsis through better diagnosis. We stand with these distinguished organizations, committed to solving sepsis and fighting antimicrobial resistance.
Sepsis Advocacy Organizations
Antimicrobial Resistance Advocacy Organizations
- HCUPnet, Healthcare Cost and Utilization Project. Agency for Healthcare Research and Quality, Rockville, MD.
- Prescott H.C., et al., Improving Sepsis Treatment by Embracing Diagnostic Uncertainty. Ann Am Thorac Soc. 2019 Apr;16(4):426-429. doi: 10.1513/AnnalsATS.201809-646PS. PMID: 30883190; PMCID: PMC6441693.
- Kellum, JA, et.al., Subtypes and Mimics of Sepsis, Critical Care Clinics, Volume 38:2, p 195-211, April 2022.
- Lengquist M et al., Sepsis mimics among presumed sepsis patients at intensive care admission: A retrospective observational study. Infection 2024 Jan 27; [e-pub]
- Chest Sepsis Mimics Infographic, Last updated June 27, 2023.
- Rudd, K.E., Kissoon, N., et al., The global burden of sepsis: barriers and potential solutions. Crit Care 22, 232 (2018).
- Timbrook, T. T., et. al., 2018, Assessments of Opportunities to Improve Antibiotic Prescribing in an Emergency Department: A Period Prevalence Survey: Adis Journals, doi:10.6084/m9.figshare.5828151.v1.
- Tamma PD, et al., JAMA Intern Med. 2017 Sep 1;177(9):1308–1315.
- Jain S, et. al., Community-Acquired Pneumonia Requiring Hospitalization among U.S. Adults, N Engl J Med 2015;373:415-427.
- Seymour CW et al., Time to treatment and mortality during mandated emergency care for sepsis. N Engl J Med 2017 May 21; [e-pub].
- Rhee C, Dantes R, Epstein L, et al; CDC Prevention Epicenter Program. Incidence and trends of sepsis in US hospitals using clinical vs claims data, 2009-2014. JAMA. 2017;318(13):1241-1249. doi:10.1001/jama.2017.13836
- https://www.cdc.gov/sepsis/what-is-sepsis.html#anchor_1547214418, Last reviewed: August 24, 2023.
- Sepsis, Content reviewed: September 2023
- Colburn, et al., Does This Adult Patient With Suspected Bacteremia Require Blood Cultures?. JAMA. 2012;308(5):502–511.
-
- Snoek, J., et al., Practical Bayesian Optimization of Machine Learning Algorithms, NeurIPS Proceedings NIPS 2018.
- Li et al., 2018 Hyperband: A Novel Bandit-Based Approach to Hyperparameter Optimization, Journal of Machine Learning Research 18 (2018) 1-52.